How solving classic probability problems can help computer scientists find bugs
In automated software testing, computer scientists employ automatic tools such as fuzzers to detect security flaws. But how long should the fuzzers test the software to guarantee that there are no bugs? Scientists at MPI-SP come up with a mathematical calculation based on probabilities.
A fuzzer is an automated test generator: it generates artificial executions of parts of the given software to detect potential vulnerabilities to attacks. The fuzzer provides random, unexpected data to see how the computer program reacts. While this technique is significantly faster compared to other methods due to its automation, finding no bugs after repeated testing does not provide a complete guarantee that no bug is present. “In empirical program analysis, we often wonder about the probability of observing a program behavior that has never previously been observed. Our approach allows us to quantify the unobserved.” Says Marcel Böhme, a faculty member at MPI-SP.
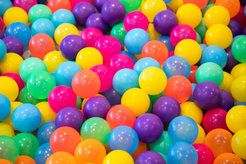
To quantify the likelihood that a new, never-seen-before behavior of a computer program will occur, the scientists at MPI-SP go back to a basic probability problem: in an urn, there is a large number of balls of many different colors (you don’t know the distribution of the colors). You extract from the urn one ball at a time. What is the probability that at the Nth extraction, you extract a ball of a color you had never extracted before? In computer science terms, what is the probability that at the Nth test, the fuzzer can detect a new, never-before-seen behavior?
“The central contribution of our work is to precisely quantify how far we can go in estimating the probability of unseen events based on observed data.” explains Seongmin Lee, a postdoctoral researcher at MPI-SP. While the Good-Turing estimator is a well-known solution to this problem, it assumes a certain type of probability model that doesn’t fit the typical software behavior space. The MPI-SP team developed a new estimator that is significantly less biased under the Multinomial model, which better reflects how software testing investigates program behaviors. “Our theory provides a way to design estimators that are tailored to a specific distribution while still being grounded in a general, distribution-free framework.”, says Lee.
The scientists published their estimator at the Thirteenth International Conference on Learning Representations in Singapore. The work was recognized as a “Spotlight” for the event.